AI algorithm can accurately tell the difference between benign and malignant breast lesions
According to a web-exclusive paper in the August issue of the American Journal of Roentgenology, during studies using breast conebeam CT (CBCT), an AI algorithm can accurately tell the difference between benign and malignant breast lesions. This has the potential to upgrade radiologist performance when deciphering the CBCT images.
The study was held at the University Medical Center Goettingen in Germany and led by Dr. Johannes Uhlig. His team trained five different machine-learning techniques in order to predict malignancy on breast legions found during CBCT breast scans. They found that the best method was the back propagation neural networks (BPNs). This method had a slightly lower sensitivity than the most experienced radiologist working during the study. However, it was more sensitive than the second radiologist working in the study, who was less experienced in CBCT. Especially of note was the fact that the algorithm was able to perform better than both readers, producing higher specificity and area under the curve (AUC).
“Once breast CBCT is clinically implemented, machine learning techniques could aid radiologists without in-depth experience in breast CBCT in image assessment,” the study said.
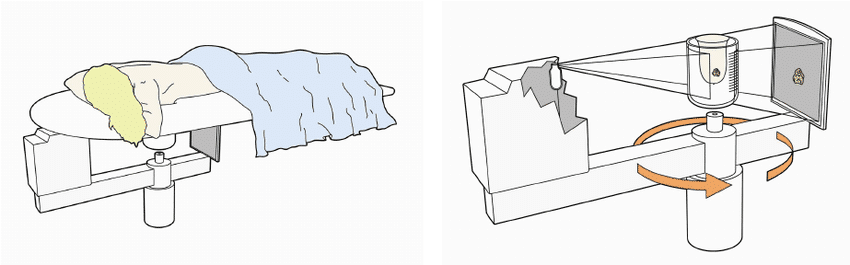
According to the authors, detecting breast lesions has been an area of exceptional quality for breast CBCT, though its ability to determine the differences between benign and malignant lesions has varied across studies in the literature.
The authors noted that “Some of this variability could be attributable to differences in reader experience with breast CBCT or divergent patient cohorts. Still, the critical question about the true diagnostic performance of breast CBCT remains, especially considering the high breast CBCT radiation dose of up to 16.6 mGy and the possibility of using alternative imaging modalities, such as MRI.”
Get Started
Request Pricing Today!
We’re here to help! Simply fill out the form to tell us a bit about your project. We’ll contact you to set up a conversation so we can discuss how we can best meet your needs. Thank you for considering us!
Great support & services
Save time and energy
Peace of mind
Risk reduction
In light of this, researchers set out to investigate machine-learning algorithms further, to determine how well they would be at predicting malignant breast CBCT lesions, and to compare their findings with human readers. Five different machine-learning techniques were trained and evaluated by the researchers. BPN, extreme learning machines, support vector machines, and K-nearest neighbors were used on a clinical dataset of 35 patients with Bl-RADS density type C and D breasts with 81 suspicious breast lesions found on contrast-enhanced breast CBCT.
10 years of breast imaging experience and 2 years of experience in dedicated breast CT were the qualifications for the two human readers used in the study, who both read the exams independently. The researchers used an internal 10-fold-cross-validation approach in order to test the machine-learning methods. This cross-validation approach evaluated many alternate versions of the available data.
For both radiologists, the difference in AUC between the machine-learning algorithm was statistically significant. For radiologist 1 (p = 0.01) and for radiologist 2 (p < 0.001). Though both readers had extensive experience in breast imaging and breast CBCT, it was noted that radiologist 1 was a bit more experienced in breast CBCT.
“Thus, in clinical practice, the diagnostic performance of general radiologists unacquainted with breast CBCT is expected to be even lower and the superiority of machine-learning techniques might be more pronounced in reality than in this study,” they wrote.
Source: https://www.ajronline.org/doi/abs/10.2214/AJR.17.19298