AI can assist radiologists in interpreting routine chest X-rays, but is unable to fully take over the radiologist’s
Though we continually hear rumors that AI will take over the radiology industry, according to new research there’s some good news for those that worry. According to the research, published on October 4 in PLOS One, AI can assist radiologists in interpreting routine chest X-Rays, as well as looking for changes on serial studies, but is unable to fully take over the radiologistβs role in reading the exams.
The research was led by Dr. Ramandeep Singh of Massachusetts General Hospital, and tested a commercial deep-learning algorithm for its ability to detect four findings on chest X-Rays. The algorithm went up against two experienced thoracic subspecialty radiologists, and it performed comparably to consensus readings and was also as accurate as independent interpretations by four other thoracic subspecialty radiologists.
The authors wrote, however, that “though helpful in improving the accuracy of interpretation, the assessed [deep-learning] algorithm is unlikely to replace radiologists due to limitations associated with the categorization of findings (such as pulmonary opacities) and lack of interpretation for specific findings (such as lines and tubes, pneumothorax, fibrosis, pulmonary nodules, and masses).”
Get Started
Request Pricing Today!
We’re here to help! Simply fill out the form to tell us a bit about your project. We’ll contact you to set up a conversation so we can discuss how we can best meet your needs. Thank you for considering us!
Great support & services
Save time and energy
Peace of mind
Risk reduction
During the research, Singh and colleagues used the U.S. National Institutes of Health ChestX-ray8 database. Along with software developer Qure.ai, they used 874 de-identified frontal chest X-Rays from 724 adult patients. Then they applied the company’s software to detect four specific findings: pulmonary opacities, pleural effusions, hilar prominence, and enlarged cardiac silhouette.
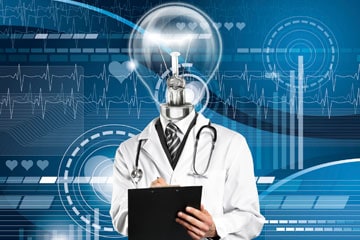
Two fellowship-trained thoracic subspecialty radiologists with 16 and 12 years of experience, respectively, established a reference standard in consensus for all 874 studies. The four other radiologists working on the research served as “test” radiologists, and independently evaluated 724 exams for the four abnormalities. Also assessed were 150 serial chest X-Rays performed as a follow-up of the initial findings.
The researchers, while they found no statistically drastic difference in accuracy between the deep-learning algorithm and the reference standing for all findings, as well as noting that the algorithm performed at least as well as the four test radiologists, they did mention there was room for improvement. Most notably in assessing change on serial exams, particularly for pulmonary opacities.
“This may have been due to variations in radiographic technique, or patient-related factors (such as differences in inspiratory effort and patient rotation over serial radiographs) on the appearance of pulmonary opacities,” the authors wrote.
It isn’t likely AI will replace radiologists, the authors noted, although the algorithm is useful for enhancing interpretation accuracy.
“The deep-learning algorithm can expedite image interpretation in emergent situations where a trained radiologist is either unavailable or overburdened in busy clinical practices,β the authors concluded. βIt may also serve as a second reader for radiologists to improve their accuracy.β